1. Introduction
- Goal
Instance를 입혀 부분적으로 Style Transfer를 해보자
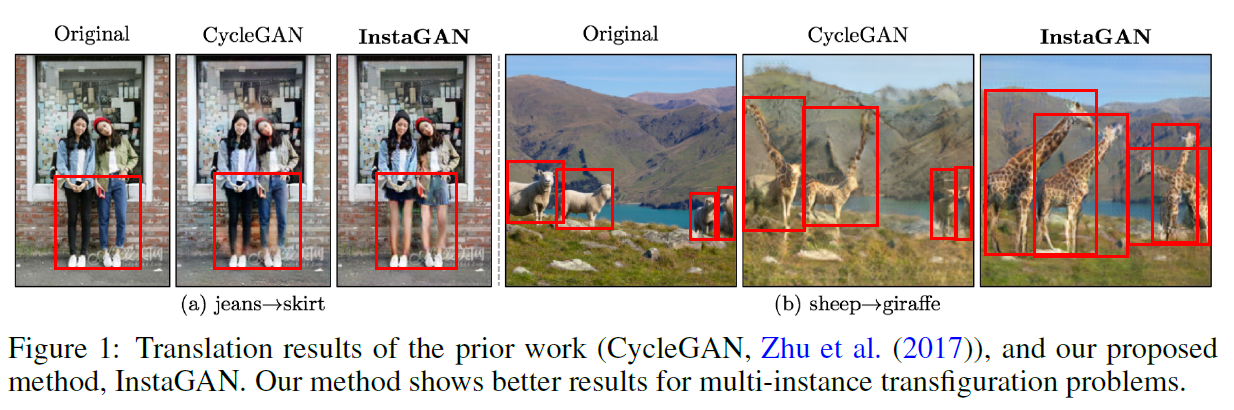
- Main Contribution
i. Image와 Instance를 모두 translate 할 수 있는 전체적인 Architecture 제안
ii. Target을 translate 할 동안 background는 모두 preserve 할 수 있는 loss를 제안
iii. mini-batch training technique를 제안
- Key
a. Disentagle b. composing appearance and shape information
- Goal
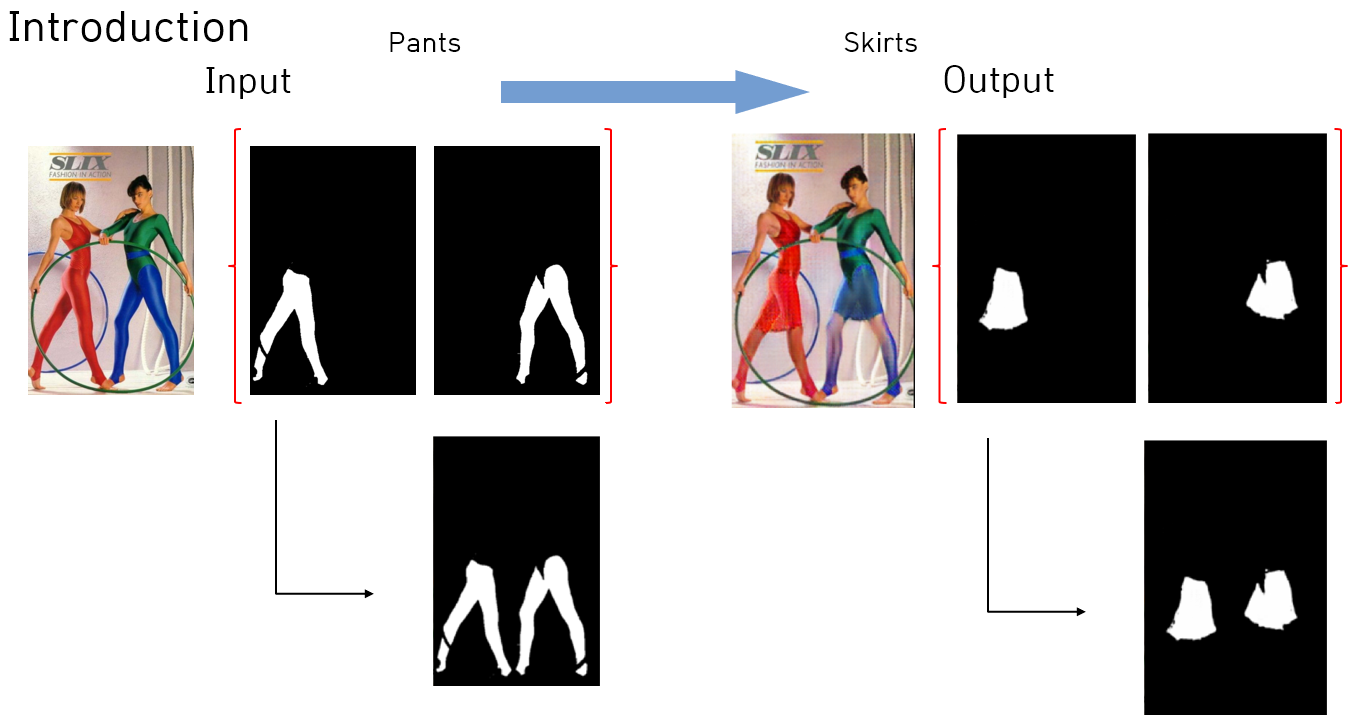
- 이 모델의 주요 Goal은 각 도메인의 Image 뿐만 아니라 Instance Information까지 함께 Cycle-GAN을 이용하여 Style Transfer하는 것.
- image2 를 보면 이해가 쉬울듯
- Benchmark Dataset
MS Coco |
---|
![]() |
MHP(Multi-human parsing) |
---|
![]() |
CCP(Clothing-co-parsing) |
---|
![]() |
- 모두 Sementic segmentation을 친절하게 제공해주는 dataset
3. Architecture
- Overview
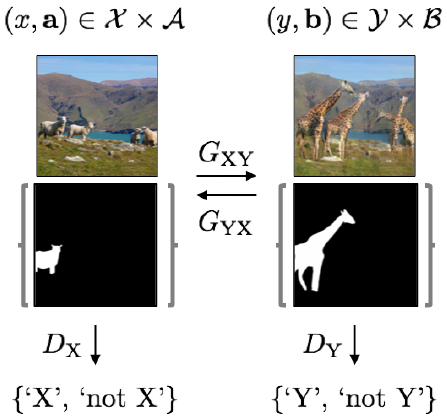
- 전체적인 overview는 CycleGAN에 꽁지(Instance Information : 이하 Inst) 가 달려있음
- Inst는 image와 마찬가지로 Cyclegan을 타고 Style-transfer를 하며 변하고자 하는 형태의 Inst로 변한다.
- 수식에서 자세히 설명
a. Generator
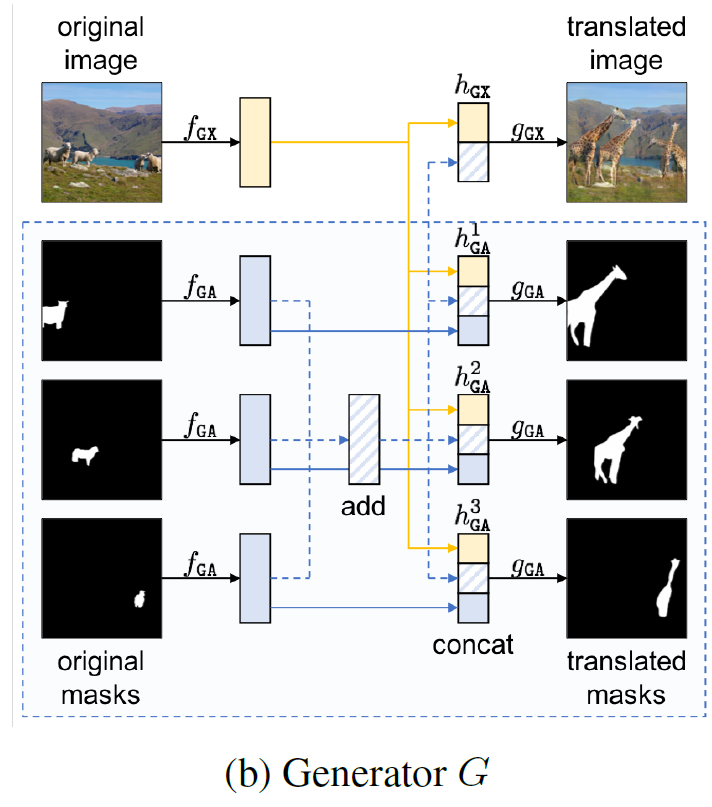
- 상당히 복잡한 구조로 이루어서 Sequential 하게 진행한다.